Journal of Credit Risk
ISSN:
1744-6619 (print)
1755-9723 (online)
Editor-in-chief: Linda Allen and Jens Hilscher
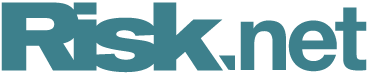
Parameterizing credit risk models
Alfred Hamerle, Daniel Rösch
Abstract
ABSTRACT
The present paper shows how the parameters of three popular portfolio credit risk models can be empirically estimated by banks using a Maximum Likelihood framework. We apply the method to a database of German firms provided by Deutsche Bundesbank and analyze the inclusion of macroeconomic and borrower specific rating factors. Given the uniform ML estimation methodology, we compare the parameter estimates and the forecast loss distributions for the credit risk models and find that they perform in very similar ways, in contrast to the differences found in some previous studies. We also propose an approach for addressing estimation errors. Our findings suggest that for a financial institution “model risk”, ie, the risk of choosing the “wrong” credit model, may be considerably reduced.
Copyright Infopro Digital Limited. All rights reserved.
As outlined in our terms and conditions, https://www.infopro-digital.com/terms-and-conditions/subscriptions/ (point 2.4), printing is limited to a single copy.
If you would like to purchase additional rights please email info@risk.net
Copyright Infopro Digital Limited. All rights reserved.
You may share this content using our article tools. As outlined in our terms and conditions, https://www.infopro-digital.com/terms-and-conditions/subscriptions/ (clause 2.4), an Authorised User may only make one copy of the materials for their own personal use. You must also comply with the restrictions in clause 2.5.
If you would like to purchase additional rights please email info@risk.net