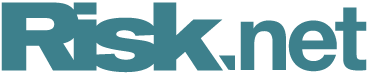
The determinants of corporate credit spreads
Credit default swaps (CDSs) are an integral tool used for the management of credit risk by financial institutions. Despite their importance, good models for the determination of CDS spreads, also called corporate credit spreads, are not readily available. Robert Jarrow, Li Li, Mark Mesler and Donald van Deventer provide such a model
Estimating credit spreads is an essential component in marking-to-market a financial institution's fixed-income investment portfolio. Credit spreads can be estimated using either bond prices, as in Campbell & Taksler (2003), Collin-Dufresne, Goldstein & Martin (2001) and Elton, et al. (2001), or using credit default swap (CDS) spreads, as in Longstaff, Mithal & Neis (2005) and Ericsson, Jacobs & Oviedo (2007). Of the two estimation procedures, the CDS-based estimates may be preferred because of the greater liquidity of the CDS markets. CDS markets are viewed as more liquid than bond markets due to the facts that (unlike trading bonds) CDSs do not require any upfront investment and enable one to more easily short a firm's credit risk. Generally speaking, there are two methods available to estimate CDS spreads.
The first approach to estimating CDS spreads is to build an analytic model, either using a structural or a reduced-form model, as for example in Jarrow & Yildirim (2004). For a review and comparison of structural and reduced-form models, see Jarrow & Protter (2004). Both approaches require specifying a stochastic process for the firm's default process, estimating the process' parameters and fitting the model to CDS spreads. If the wrong stochastic process is selected, then the model will be mis-specified and the CDS model estimates biased. At this stage in the literature, there is still no consensus concerning the best stochastic process to model defaults (structural or reduced-form). Indeed, this difference of opinions can be most easily seen in the recent debate concerning how best to model a firm's default correlation. In addition, the standard analytic CDS models assume friction-less and perfectly liquid markets. And, although more liquid than bond markets, CDS markets have still not reached this theoretical abstraction. The friction-less and perfectly liquid assumptions induce another model mis-specification. Unfortunately, incorporating market frictions and or market illiquidities into these analytic structures is an unresolved and, at best, difficult task (see Jarrow & Protter (2005) for a review).
The second approach to estimating CDS spreads is to fit a statistical model. Although not as conceptually satisfying as an analytic model, a statistical model is useful when constructing an analytic model is too complex. At present, this is the case for modelling CDS spreads using either a structural or reduced-form model. Fortunately, a statistical model can be thought of as an approximation to the more general, yet unspecified analytic model. This insight provides a more satisfying justification for using a statistical model. Indeed, it is well known that linear regression analysis (itself a statistical model) can be viewed as providing a linear approximation to a more complex and non-linear economic model.
Based on this justification, the purpose of this article is to provide a statistical model for fitting CDS spreads. Our statistical model uses explanatory variables intended to characterise the essential components of credit risk - default probabilities and recovery rates. The estimated model provides a good fit to CDS spreads, with an ^R2 equal to 80%. Yet, a significant portion of CDS spreads remains unexplained. This omitted component supports the contention that market liquidities and microstructure effects are still an important and not well-understood considerations in the determination of CDS spreads.
Our CDS statistical model has various potential uses. First, it can be used to determine CDS spreads for thinly traded credit entities. Indeed, given that the relevant independent variable inputs can be obtained for the reference entity, the statistical model gives a forecast CDS spread. Second, our statistical model can also be used to determine market mis-pricings in traded CDSs. Indeed, the statistical model is one way to determine a 'fair' CDS spread, which can be compared with actual market quotes.
The following section provides the statistical model, then we discuss the data and estimation procedure, while the last section concludes.
1 The one-year CDS maturity is omitted to avoid having the independent variable matrix singular.
2 There is government assistance in the form of the Industrial Revitalization Corporation of Japan. In addition, the banking system often results in the 'main lending bank' to a key corporation in its industrial group taking losses in the same way that a subordinated lender would, even though the bank loan is senior and nominally pari passu with other senior lenders. There is anecdotal evidence that non-bank lenders expect the main banks to act in this way, and such lenders accept lower spreads because of it, even though it is not explicit in the documentation of the credit.
REFERENCES
Campbell ^J and G Taksler, 2003
Equity volatility and corporate bond yields
Journal of Finance 58 (6), December, pages 2,321-2,349
Collin-Dufresne P, R Goldstein and ^J Martin, 2001
The determinants of credit spread changes
Journal of Finance 56 (6), December, pages 2,177-2,207
Elton E, M Gruber, D Agrawal and C Mann, 2001
Explaining the rate spread on corporate bonds
Journal of Finance 56 (1), February, pages 247-277
Ericsson J, K Jacobs and R Oviedo, 2007
The determinants of credit default swap premia
Journal of Financial and Quantitative Analysis
Jarrow R and S Chava, 2004
Bankruptcy prediction with industry effects
Review of Finance 8 (4), pages 537-569
Jarrow R and ^P Protter, 2004
Structural versus reduced form models: a new information based perspective
Journal of Investment Management 2 (2), second quarter, pages 1-10
Jarrow R and ^P Protter, 2005
Liquidity risk and option pricing theory
Handbook of Financial Engineering, Elsevier Publishers
Jarrow R and Y Yildirim, 2004
A simple model for valuing default swaps when both market and credit risk are correlated
Journal of Fixed Income, March, pages 1-19
Longstaff F, S Mithal and E Neis, 2005
Corporate yield spreads: default risk or liquidity? New evidence from the credit default swap market
Journal of Finance 60 (5), October, pages 2,213-2,253
Maddala G, 1992
Introduction to econometrics
Second edition, Prentice Hall
3 The t-statistic and not the Wald ratios are appropriate given our transformation from expression (1) to (2). The t-statistic is the coefficient divided by the standard error. Under the standard ordinary least squares (OLS) assumptions, it provides a method to determine the significance of the independent explanatory variables. Given that we estimate a pooled time series and cross-sectional regression, the standard OLS (independent residuals) assumption is probably not satisfied. Consequently, the t-statistics should only be viewed as approximate.
Only users who have a paid subscription or are part of a corporate subscription are able to print or copy content.
To access these options, along with all other subscription benefits, please contact info@risk.net or view our subscription options here: http://subscriptions.risk.net/subscribe
You are currently unable to print this content. Please contact info@risk.net to find out more.
You are currently unable to copy this content. Please contact info@risk.net to find out more.
Copyright Infopro Digital Limited. All rights reserved.
You may share this content using our article tools. Printing this content is for the sole use of the Authorised User (named subscriber), as outlined in our terms and conditions - https://www.infopro-insight.com/terms-conditions/insight-subscriptions/
If you would like to purchase additional rights please email info@risk.net
Copyright Infopro Digital Limited. All rights reserved.
You may share this content using our article tools. Copying this content is for the sole use of the Authorised User (named subscriber), as outlined in our terms and conditions - https://www.infopro-insight.com/terms-conditions/insight-subscriptions/
If you would like to purchase additional rights please email info@risk.net
More on Credit markets
Liquidnet sees electronic future for grey bond trading
TP Icap’s grey market bond trading unit has more than doubled transactions in the first quarter of 2024
Single-name CDS trading bounces back
Volumes are up as Covid-driven support fuels opportunity for traders and investors
Podcast: Richard Martin on improving credit migration models
Star quant proposes a new model for predicting changes in bond ratings
CME to pass on Ice CDS administration charges
Clearing house to hike CDS index trade fees from July after Ice’s determinations committee takeover
Buy side fuels boom in single-name CDS clearing
Ice single-name CDS volumes double year on year following switch to semi-annual rolls
Ice to clear single-name bank CDSs from April 10
US participants will be able to start clearing CDSs referencing Ice clearing members
iHeart CDS saga sparks debate over credit rules
Trigger decision highlights product's weaknesses, warns Milbank’s Williams
TLAC-driven CDS index change tipped for September
UK and Swiss bank Holdco CDSs likely inclusions in next iTraxx index roll, say strategists