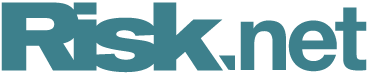
Credit tails
Models have taken a lot of flak in the past few months, with dealers and investors alike racking up millions of dollars in losses after the second major dislocation in the credit markets in two years. Jon Gregory considers some of the problems with credit models
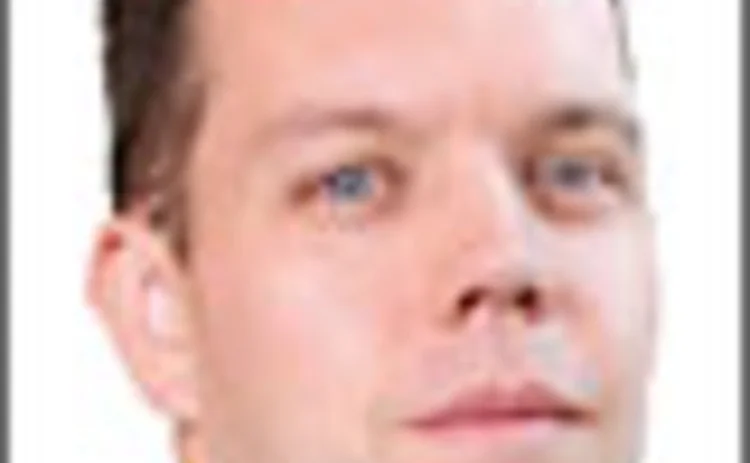
When times are good, investors take on more risk. When the cycle turns, they offload this risk. The ensuing flight to quality may cause a gradual market downturn or, more likely, lead to a collapse. This description could be applied in general to a financial crisis, but could also be used to describe the recent meltdown in the US subprime mortgage market.
The surge in US subprime delinquencies redefined the market perception of mortgage risk, and this quickly spread to other sectors of the credit markets. Assets have fallen sharply in value and volatility has increased, while the commercial paper market has seized up, making the rollover of short-term obligations by banks, conduits and structured investment vehicles (SIVs) impossible.
Looking back, risk premiums hit a record low back at the beginning of 2007 across a broad variety of assets. Credit spreads had ground to historic tight levels, and innovation and complexity were running rampant in order to create (high leverage) structures to generate enhanced returns. Investor appetite was strong and there was little discrimination between different types of vehicles. This combination of innovation and high leverage, difficulties in pricing and risk management of these structures, and the opaque nature of some of the products have formed a nasty cocktail with a rather unpleasant and potentially long-lasting hangover.
As with the May 2005 correlation crisis, a lot of attention has focused on financial models and their potential misuse in the credit world. Is the crisis the fault of the financial engineers who spend their days thinking about stochastic processes, default correlation and extreme value theory? Are the rating agencies to blame for using evermore complex models to assign ratings to highly leveraged products? Have banks been naively taking liquidity and gap risk under the assumption that certain events will not happen?
This article aims to highlight some of the problems with credit models and offer some solutions. I will argue that the structured credit market is suffering from the same problems that have existed in other, now more mature areas, but has been magnified by the explosive growth of credit derivatives. At the core of the problem is that structured credit is defined by relatively rare credit events and co-dependency that is hard to characterise and measure. But I will also suggest that, in the credit arena especially, we must look far beyond the actual models to their use, intended or otherwise, and the impact they can have on the market.
This article will make a broad division between objective and risk-neutral models. The former category refers to economic representations defining a rating or mark-to-model price via subjective assessment of credit risk (default probability and risk premium). Risk-neutral models, on the other hand, are used under the implicit assumption that there exists some underlying hedging strategy to justify the price.
Objective models
This term can broadly cover three areas:
- Rating agency models.
- Mark-to-model approaches, which are used by investors to price products that are illiquid.
- Gap risk models, which are used by issuers to assess the price of the gap risk they retain when issuing structures such as constant proportion portfolio insurance.
One can argue that rating agencies have incentives to produce evermore complex models as they are effectively paid for assigning ratings to structured credit products. The rating agency modelling approach can be broadly summarised as the design of an economically motivated model, parameterised with historical data, which is then applied to the product cashflows to produce a single measure of risk (typically expected loss or probability of default), mapped to a rating. It should be fairly obvious that rating agencies are making an assessment of the risk of not receiving promised returns on a single product under the assumption that history provides a reasonable prediction of the future. There is a big difference between creditworthiness, which the rating agencies attempt to quantify, and market price, which they do not.
The rating process for synthetic collateralised debt obligations (CDOs) is relatively transparent, but things become trickier when we consider more complex structures. For instance, a dynamic leverage structure such as a constant proportion debt obligation (CPDO) should ultimately overcome losses from roll costs, spread widening and defaults as it is a long-maturity product. Increased volatility is therefore a good thing, so long as the CPDO does not cash out (that is, hit 10% of its initial value, prompting the product to liquidate automatically). But the difference between a CPDO that eventually returns full coupons and principal after a stormy ride and one that completely deleverages in its early years may be rather subtle. The rating models required for such structures are, of necessity, an order of magnitude more complex and involve spread process assumptions (volatility, mean reversion and even explosiveness), and this creates greater uncertainty in the rating process.
It is not just rating agencies that rely on objective models. Banks also use complex models to assess gap risk on CPDOs and leveraged super-senior (LSS) tranches they have issued. Meanwhile, many investors rely on their own proprietary techniques to mark-to-model illiquid products using non-observable inputs. There are challenges to such an approach. When valuing a residential mortgage-backed securities tranche, for example, one cannot assume the deal would be wiped out by a single event, but one cannot model each mortgage in the underlying portfolio individually. Likewise, pricing a tranche of a cashflow CDO is difficult due to the complex structure of the underlying product and the presence of waterfalls and detailed rules regarding diversion of cashflows.
Nevertheless, none of these technical points are a worry for the average quant, well versed in stochastic calculus and commanding a vast array of statistical, mathematical and numerical skills. A well-chosen range of tools from the quant's toolbox will lead to the development of a model that can capture the key components, parameterised with historical data and used to predict the future.
However, models may cause a false sense of security by attaching a low probability to the very events that should concern us. For example, a model may not capture the reality that more than half the widening of an index can be apportioned to a relatively small number of names1 (potentially crucial for assessing CPDO roll cost) or that super-senior correlation may approach 100%, driven by supply and demand.
As Ockham's razor2 (paraphrased) states: "All things being equal, the simplest solution tends to be the best one." Perhaps the Einstein quote (again paraphrased) of "theories should be as simple as possible, but no simpler" is more accurate. Objective models are overly complex, make unrealistic modelling assumptions and rely on limited data not representative of the future. Simpler and more transparent approaches will be more easily understood, less open to abuse and clearer in their limitations. Rapid growth should not be achieved by overreliance on quantitative models, but should include potentially more time-consuming qualitative assessments.
By way of illustration, consider the application of value-at-risk (VAR) models in market risk management that calculate a metric for the portfolio in question (for example, a 99th percentile for a 10-day horizon). Such a model can be empirically back-tested by calculating the number of times VAR was actually exceeded.3 On this basis, the model might be reasonably accurate4 - not bad given the relatively complex multidimensional nature of most derivatives portfolios.
However, AAA rated structured credit products require dealing with event probabilities more in line with once in many hundreds of years. If an AAA tranche priced at $99.50 takes a loss in year one, then it will not be possible to appreciate in one's lifetime whether this is just bad luck or a catastrophic failure of the rating and mark-to-model approach. Even investing in many products and relying on the law of large numbers will not help, as no claim is made that the individual products will behave independently of one another (indeed, quite the reverse should be expected).
In summary, objective models can be over-complex, poorly parameterised and rely on imperfect distributional assumptions - and produce a single statistical measure that cannot be empirically tested. If such models are developed with intuition and flair, they may add some value for understanding and comparing rather complex risks with reference to some baseline. But one must remember that 10-sigma events, unknown unknowns, Black Swans - call them what you will - are not predicted by studying historical data and using normal distributions, mean reversions, credit migration probabilities and correlations. Since we care about rare events, a good or bad model will be defined less by the assumptions made and more by the context in which it is used. In the context of structured credit, over-use of a good model can be worse than under-use of a poor one.
Self-negating prophecies
Ideas in the financial world are copied - structured products cannot be patent protected - and good ideas are reproduced and modified quickly, leading to a huge volume of effectively the same product hitting the market in a relatively short space of time. This may negate the very assumptions that led to the development of the product in the first place.
For instance, in an SIV or SIV-lite structure, the high leverage amplifies movements in the net asset value (NAV) of the portfolio. A breach of a market value trigger, which occurs when the NAV falls below a certain level, will lead to operating restrictions being imposed and may force the SIV to sell assets. This, in turn, is likely to have a negative effect on other SIVs via price pressure and funding issues.
The same thing can occur on LSS transactions, which have an additional risk factor of the super-senior correlation level in the event of unwind. A key driving force in determining super-senior correlation then becomes the amount of LSS issued.5
This self-negating prophecy is not specific to credit. Traditional portfolio insurance, a technique of automated selling designed to limit downside exposure to the stock market, instead acted as an accelerant in the 1987 crash due to similar price pressure arguments as above.
Another problem one might identify is the difference between correlation and skew in the market and that used by rating agencies. In a sell-off, high correlations may be created, and this may put pressure on rating agencies to revise historical correlation estimates. Similar occurrences are seen in other markets - for example, implied volatility can drive historical volatility (rather than the other way around, as is often assumed) as a result of rapid delta hedging in volatile conditions.
Risk-neutral models
So far, I have discussed the weaknesses of the naive use of pricing approaches implemented via over-complicated models. On the other hand, the appeal of risk-neutral valuation is that the price can be justified by reference to a replication strategy achievable via dynamic hedging. Dynamic hedging is a theoretical ideal that is more challenging in practice, especially for credit. However, models of this type are invaluable since, in normal market conditions, they tell you how to hedge via neutralising first-order moves. This unfortunately means risk management of synthetic CDOs has generally been based on assumptions of first-order moves and simple correlation measures, and much less on actual experience.
If you search for criticism of the Gaussian copula model and associated base correlation approach, one practical illustration of its failure is often demonstrated via the variation in tranche deltas. An example often given is of buying equity protection and delta hedging by selling protection on the index. A significant widening of spreads will typically cause the delta of the equity tranche to decrease and so creates an unpleasant negative gamma (buying back index protection at higher premiums).
This situation can be even worse if the equity is hedged with another tranche (for instance, the junior mezzanine) since a spread widening accompanied by steepening of the correlation curve means the hedge can magnify the loss. Failed CDO delta hedges may then fuel further market volatility.
Using such an example to illustrate the weakness of the model misses the point somewhat - delta does not hedge anything except a small move in one underlying. For larger moves, gamma and cross-gamma (in the latter case, the dependence of moves between correlation and spreads) play a key role.
This phenomenon is not specific to credit. Hedging the interest rate risk of a swaption with a swap depends on the behaviour of swap rates and volatility. For extreme moves, this may not be obvious and can also be affected by supply and demand, potentially leading to the view that the model's delta is wrong. Just as we might argue that current credit models have performed badly in 2005 and 2007, we can point to similar problems regarding the Bermudan swaptions approaches in the 1990s. In credit, the problem is magnified as spreads can readily double or even halve in a relatively short space of time (whereas moves of similar proportions in interest rates, equities, foreign exchange and commodities are less likely), and also because correlation can both increase or decrease the value of a tranche (a mezzanine tranche may not be monotonic in a certain range and can therefore go from short correlation to long correlation if spreads move sufficiently).
The current market-standard approach is criticised in many ways, often connected with the presence of arbitrage in prices. But all these points should be secondary to the assessment of the hedging capabilities of the model. If the model is tractable and fits the market perfectly, then the ability to calculate hedges and explain profit and loss variations might be rather good. Improved models are typically accompanied by better hedging strategies. Why not understand fully the problems with the hedging in the current model before anything is changed?
The role of accounting
Base correlation provides an appealing way to price bespoke CDO tranches with reference to traded index tranches. The use of a practical measure such as correlation in this linkage is intuitive, but a rather steep correlation skew is required to fit the market. A direct implication of working with a non-flat correlation curve is that when pricing bespoke portfolios, and more importantly calculating deltas and other Greeks, we have to decide how to factor in changes in correlation.
Herein lays the main issue: since the correlation curve is far from flat, the calculation of a credit delta requires some heroic assumptions about the behaviour of correlation with respect to spread moves. Sometimes, the correlation contribution to the delta is greater than the spread contribution. This is bad - a delta hedge should not be a correlation hedge. Hence, there is clearly some significant benefit in having a model with a flat correlation smile - that is, one that fits the market prices. Given the amount of effort that has been put into finding such an approach (see Burtschell, Gregory & Laurent (2005) and Ferrarese (2006)), why has the Gaussian copula approach persisted?
Accounting changes have played a key role in shaping today's derivatives markets. Initiatives such as International Accounting Standard 39 (which covers financial reporting standards for financial instruments) means banks are forced to mark-to-market rather than mark-to-model. The price is the price, whether or not it is irrational and incompatible with a model. One of the results of this is that prices are not as model-driven as they were a decade ago. Supply and demand is the dominant factor in explaining prices, and risk-neutral models are not there to express a plausible view - they are there to interpolate and extrapolate market prices.
Approaches such as base correlation and Gaussian copula work well because they can be easily calibrated to the market. The market is driven by a strange force that equates supply and demand, and the dynamics of such a force are not easy to capture in a few equations. Not surprisingly, the market has stuck to an approach that is heavily flawed but fits prices perfectly.
A problem with this accounting-driven approach to model development is that there is less incentive to produce good models based on fundamentals and robust theoretical constructions. A model with enough flexibility can be made to match market prices, without necessarily capturing reality in any significant way. This may explain why there has been a huge amount of interest in pricing structured credit derivatives, but very little associated research on hedging implications.6 This would seem to be an obvious point in any asset class, but is particularly critical in credit due to the discontinuities in underlying variables and the arbitrary pricing methods applied to CDOs.
Of course, any new model can be assessed in different ways, such as its dynamics, arbitrage-free characteristics, pricing of non-standard products and the ability to reproduce market prices. But the ultimate test of a CDO model should be its ability to actually provide a good practical hedging strategy. Recent approaches (such as Baxter (2007) and Inglis & Lipton (2007)) address some of the core pricing issues with the base correlation/Gaussian copula approach, but they represent quite a big step in terms of the hedging of CDOs since the underlying hedges will be based upon new model parameters. An approach such as that of Garcia, et al. (2007) would represent a smaller step forward in terms of a reasonably simple way in which to flatten base correlation curves, and should not be rejected on the basis that it does not represent a major change of direction or complete solution.
Where do we go from here?
For the average investor, a single measure (rating) is much easier to understand than a multidimensional one, but there are many fundamental problems with representation of credit risk via a single measure. In a similar vein, while objective models are useful for mark-to-model pricing or assessment of gap risk, the price is bound to mislead and cause false comfort. The problem is not that models ignore liquidity, but rather that market participants ignore liquidity by relying on single measures to summarise what is rather complex. Such practices and lack of understanding of complex modelling approaches can create an asymmetric information problem, meaning sudden price changes, not entirely driven by news, are conceivable.
While models can improve understanding and transfer of risk, they can also act as self-negating prophecies and magnify market downturns. A key driving force in determining super-senior correlation is the amount of LSS tranches issued. The tipping point at which we transgress from positive to negative model behaviour may be hard to assess. It might be that, by assuming excessive randomness, models may help to avoid such extreme market situations. This would clearly equate to being overly conservative about objective pricing or assigning AAA ratings to complex risk.
Although tranching is a powerful method for efficient distribution of risk, it is also a complex procedure, requiring structurers, rating agencies, trustees, lawyers and accountants, and can ultimately lead to a misrepresentation of risk versus return. Investors should demand increased transparency on complex financial products and not rely too heavily on the ratings and mark-to-model prices of structured credit products such as CDOs and CPDOs to make investment decisions.
While the use of objective models for pricing of complex products has been widespread in credit, risk-neutral approaches (where the concept of price is actually theoretically justified) are hindered. Marking-to-market and risk management are inextricably linked, yet are partly driven apart by regulatory requirements. There is no right model to explain market prices in a technical market. Any model that fits the market precisely is likely to beat ones that do not. There should be renewed effort to develop good models that will be judged on their risk management abilities. Since the very nature of credit gives rise to partially unhedgeable risks, it is also important to consider non-linearities - for example using scenario analysis.
In theory, repricing in the credit markets should mean there is less pressure for complexity and leverage. On the other hand, innovation may be needed to produce products with certain characteristics (such as low price volatility) that do not turn into self-negating prophecies. While I would argue the focus on objective models should be more simplistic and clear, advances in risk-neutral pricing are definitely of value for widening the range of synthetic securitisations to cover assets such as leveraged loans, asset-backed securities and commercial mortgage-backed securities and complex structures such as LSS tranches. Remember that a price is nothing without a hedging strategy. Given the choice, choose risk-neutral valuation and not an objective assessment of small probabilities.
Jon Gregory is global head of credit quantitative analytics at Barclays Capital. A longer version of this article is available from the author on request. The views and options represented in this article are solely those of the author and do not necessarily represent those of his employer. The author is grateful for comments on an initial draft from Nick Dunbar, Matthew Leeming, Donald MacKenzie and Riccardo Rebonato. Email: jon.gregory@barclayscapital.com
1 For example, almost 60% of the widening in the CDX five-year index between October 12 and November 5, 2007 came from just 10 of the 125 names. (Source: Barclays Capital Structured Credit Research.) A CPDO will roll every six months by unwinding the credit protection sold and selling new protection on the current index. Because the current index is longer-dated than the old index, it normally trades at a wider spread, which gives the CPDO extra income. However, if the new index trades inside the old index, losses are likely as it is the 'blown up' or downgraded credits that are removed from the new basket and replaced with average spread names.
2 William of Ockham, a 14th century logician.
3 From the definition of VAR (99th percentile) this equates to a 1% probably for a 10-day period so we should expect a breach around once every two years.
4 Note that this accuracy might be debated and there is still criticism of the market risk VAR concept, with some claiming it gives a false sense of security to risk managers, senior management and regulators. But such objections are typically related to misunderstandings, such that a loss of several multiples of VAR is highly improbable.
5 Most of the rating agencies have not rated market value trigger LSS contracts, which could be taken as a realisation of this point.
6 As was recently pointed out to the author by Jean-Paul Laurent, a survey of the credit modelling resource www.defaultrisk.com shows that there are around 1,000 papers on pricing but only 10 dedicated to hedging issues.
REFERENCES
Baxter M, 2007
Gamma process dynamic modelling of credit
Risk October, pages 98-101
Burtschell X, J Gregory and J-P Laurent, 2005
A comparative analysis of CDO pricing models
Working paper
Duffie D, 2007
Innovations in credit risk transfer: implications for financial stability
Graduate School of Business, Stanford University
Ferrarese C, 2006
A comparative analysis of correlation skew modeling techniques for CDO index tranches
Working paper
Garcia J, S Goossens, V Masol and W Schoutens, 2007
Levy base correlation
Working paper, available at www.schoutens.be
Inglis S and A Lipton, 2007
Factor models for credit correlation
Working paper, available at www.defaultrisk.com
MacKenzie D, 2006
An engine, not a camera: how financial models shape markets
MIT Press
Rebonato R, 2007
Plight of the fortune tellers: why we need to manage financial risk differently
Princeton University Press.
Only users who have a paid subscription or are part of a corporate subscription are able to print or copy content.
To access these options, along with all other subscription benefits, please contact info@risk.net or view our subscription options here: http://subscriptions.risk.net/subscribe
You are currently unable to print this content. Please contact info@risk.net to find out more.
You are currently unable to copy this content. Please contact info@risk.net to find out more.
Copyright Infopro Digital Limited. All rights reserved.
You may share this content using our article tools. Printing this content is for the sole use of the Authorised User (named subscriber), as outlined in our terms and conditions - https://www.infopro-insight.com/terms-conditions/insight-subscriptions/
If you would like to purchase additional rights please email info@risk.net
Copyright Infopro Digital Limited. All rights reserved.
You may share this content using our article tools. Copying this content is for the sole use of the Authorised User (named subscriber), as outlined in our terms and conditions - https://www.infopro-insight.com/terms-conditions/insight-subscriptions/
If you would like to purchase additional rights please email info@risk.net
More on Credit markets
Liquidnet sees electronic future for grey bond trading
TP Icap’s grey market bond trading unit has more than doubled transactions in the first quarter of 2024
Single-name CDS trading bounces back
Volumes are up as Covid-driven support fuels opportunity for traders and investors
Podcast: Richard Martin on improving credit migration models
Star quant proposes a new model for predicting changes in bond ratings
CME to pass on Ice CDS administration charges
Clearing house to hike CDS index trade fees from July after Ice’s determinations committee takeover
Buy side fuels boom in single-name CDS clearing
Ice single-name CDS volumes double year on year following switch to semi-annual rolls
Ice to clear single-name bank CDSs from April 10
US participants will be able to start clearing CDSs referencing Ice clearing members
iHeart CDS saga sparks debate over credit rules
Trigger decision highlights product's weaknesses, warns Milbank’s Williams
TLAC-driven CDS index change tipped for September
UK and Swiss bank Holdco CDSs likely inclusions in next iTraxx index roll, say strategists
Most read
- Basel Committee reviewing design of liquidity ratios
- Breaking out of the cells: banks’ long goodbye to spreadsheets
- Too soon to say good riddance to banks’ public enemy number one