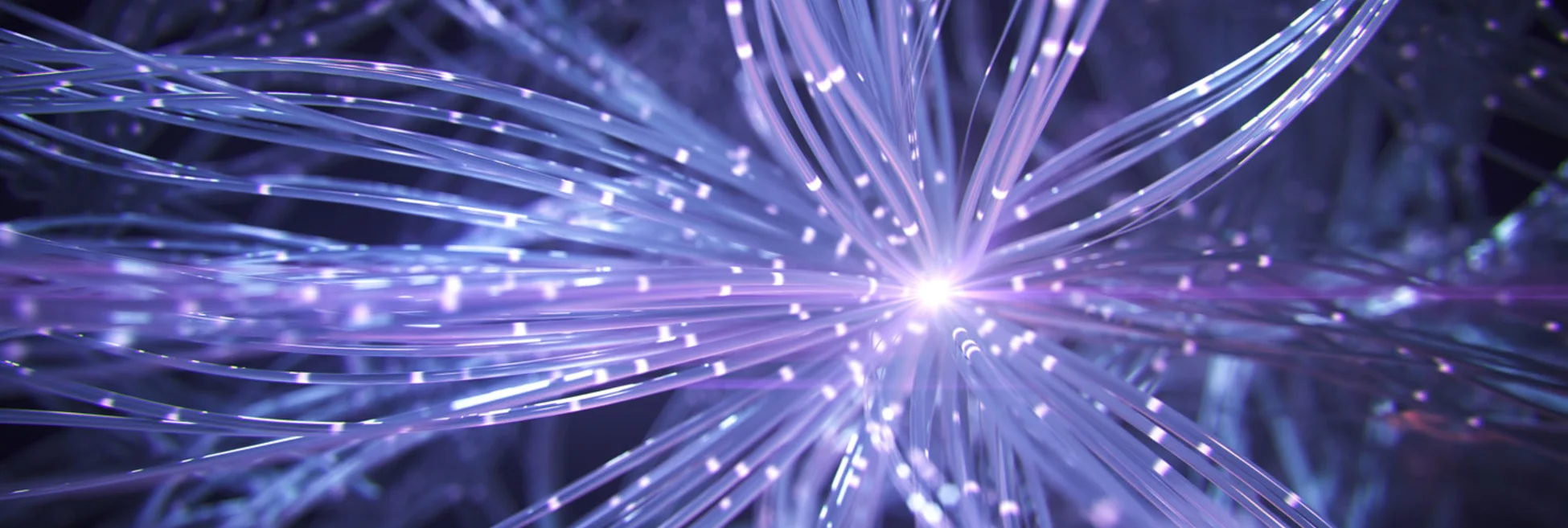
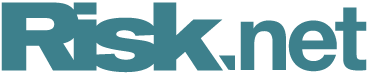
Interpretability of neural networks: a credit card default model example
Recently developed techniques aimed at answering interpretability issues in neural networks are tested and applied to a retail banking case
CLICK HERE TO VIEW THE PDF
Ksenia Ponomareva and Simone Caenazzo show the feasibility of overcoming the interpretability hurdles around the application of neural networks in the estimation of credit risk for a portfolio of credit cards
Historically, the widespread use of advanced deep learning models in sensitive fields such as medicine and finance has been hindered by a fundamental lack of interpretability regarding the outcomes of such models. Simpler techniques such as linear or logistic
Only users who have a paid subscription or are part of a corporate subscription are able to print or copy content.
To access these options, along with all other subscription benefits, please contact info@risk.net or view our subscription options here: http://subscriptions.risk.net/subscribe
You are currently unable to print this content. Please contact info@risk.net to find out more.
You are currently unable to copy this content. Please contact info@risk.net to find out more.
Copyright Infopro Digital Limited. All rights reserved.
You may share this content using our article tools. Printing this content is for the sole use of the Authorised User (named subscriber), as outlined in our terms and conditions - https://www.infopro-insight.com/terms-conditions/insight-subscriptions/
If you would like to purchase additional rights please email info@risk.net
Copyright Infopro Digital Limited. All rights reserved.
You may share this content using our article tools. Copying this content is for the sole use of the Authorised User (named subscriber), as outlined in our terms and conditions - https://www.infopro-insight.com/terms-conditions/insight-subscriptions/
If you would like to purchase additional rights please email info@risk.net
More on Banking
Volatility shape-shifters: arbitrage-free shaping of implied volatility surfaces
Manipulating implied volatility surfaces using optimal transport theory has several applications
Infrequent MtM reduces neither value-at-risk nor backtesting exceptions
Frequency of repricing impacts volatility and correlation measures
SABR convexity adjustment for an arithmetic average RFR swap
A model-independent convexity adjustment for interest rate swaps is introduced
Joint S&P 500/VIX smile calibration in discrete and continuous time
An arbitrage-free model for exotic options that captures smiles and futures is presented
The carbon equivalence principle: minimising the cost to carbon net zero
A method to align incentives with sustainability in financial markets is introduced
Leveraged wrong-way risk
A model to assess the exposure to leveraged and collateralised counterparties is presented
Neural joint S&P 500/VIX smile calibration
A one-factor stochastic local volatility model can solve the joint calibration problem