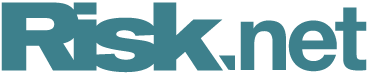
Energy firms grapple with rising customer risk
Understanding the risks a firm is exposed to through its counterparties and customers is vital in today’s world, not just from a credit perspective but for compliance and reputational reasons, writes a senior analyst. Here she shares her experience of building a sophisticated customer screening programme
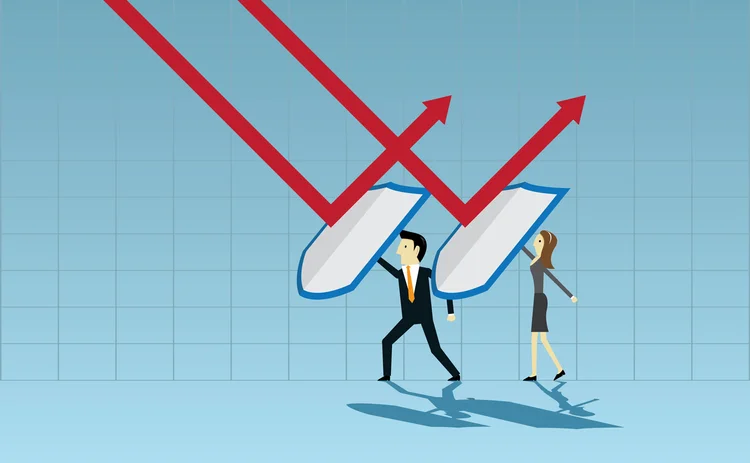
Understanding customer risk is vital in today’s environment of heightened geopolitical tensions, growing sanctions and increasing regulatory scrutiny.
It is essential that firms are able to identify and avoid counterparties and customers that might be involved in terrorism, bribery or corruption, or that are operating fraudulently or dishonestly. Given the complex interlinkages between companies globally and the range of customers that most firms have these days, customer screening is becoming
Only users who have a paid subscription or are part of a corporate subscription are able to print or copy content.
To access these options, along with all other subscription benefits, please contact info@risk.net or view our subscription options here: http://subscriptions.risk.net/subscribe
You are currently unable to print this content. Please contact info@risk.net to find out more.
You are currently unable to copy this content. Please contact info@risk.net to find out more.
Copyright Infopro Digital Limited. All rights reserved.
You may share this content using our article tools. Printing this content is for the sole use of the Authorised User (named subscriber), as outlined in our terms and conditions - https://www.infopro-insight.com/terms-conditions/insight-subscriptions/
If you would like to purchase additional rights please email info@risk.net
Copyright Infopro Digital Limited. All rights reserved.
You may share this content using our article tools. Copying this content is for the sole use of the Authorised User (named subscriber), as outlined in our terms and conditions - https://www.infopro-insight.com/terms-conditions/insight-subscriptions/
If you would like to purchase additional rights please email info@risk.net
More on Commodities
Energy Risk 2024 Software Rankings: IT demands increase amid rising risk
Heightened geopolitical and credit risk increase requirements on commodities software
Energy Risk Asia Awards 2023: the winners
Winning firms demonstrate resiliency and robust risk management amid testing times
ION Commodities: addressing the market’s recent pain points
Energy Risk Software Rankings winner’s interview: ION Commodities
Energy Risk Commodity Rankings 2023: adapting to new market dynamics
Winners of the 2023 Commodity Rankings provided reliability when clients faced extreme change
Energy Risk Software Rankings 2023: managing uncertainty
Unpredictable markets make CTRM software choices key
Navigating the volatility and complexity of commodity markets
Commodity markets have experienced significant challenges since the Covid-19 pandemic, the conflict in Ukraine and the subsequent sanctions imposed on Russia. These unprecedented events have caused fluctuations in supply and demand, disrupted global…
Energy Risk Asia Awards 2022
Recognising excellence in energy risk management
Market shrugs off EC’s plan to change gas benchmark
Dutch TTF prices unmoved, as market participants say they are “not taking it seriously”
Most read
- Breaking out of the cells: banks’ long goodbye to spreadsheets
- Too soon to say good riddance to banks’ public enemy number one
- Industry calls for major rethink of Basel III rules