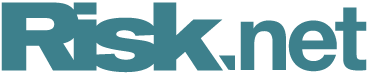
Buy-side quants of the year: Nicholas Westray and Kevin Webster
Risk Awards 2024: Debiasing technique for data could usher in golden age of transaction cost analysis
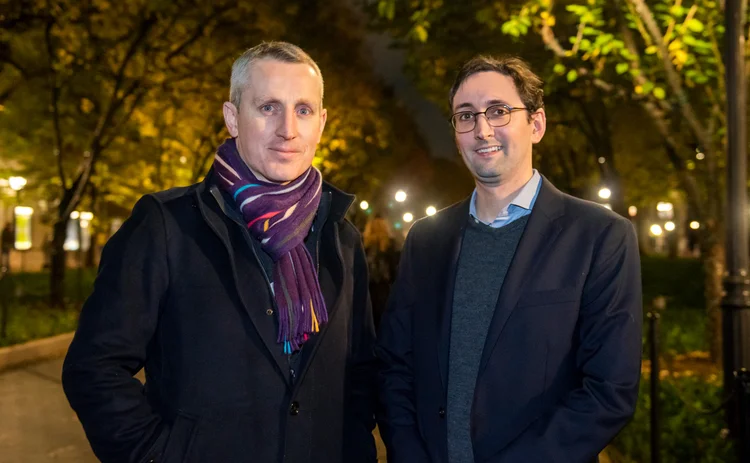
The collaboration between Nicholas Westray and Kevin Webster that led to their selection as Risk.net’s buy-side quants of the year started over an informal lunch in New York.
Webster had left Citadel, and while on gardening leave had spent some of his time watching YouTube replays of Microsoft’s annual research conference. He began to think that ideas about causal inference that were creating a buzz among Microsoft’s engineers could help solve problems in finance, too.
I told Kevin about the simulator, and he said it was exactly what he needed
Nicholas Westray
Webster formed an idea for a paper that would apply techniques from the tech world to an old problem facing investors – how to accurately measure the market impact of their own trades. But he lacked the data to test whether the idea worked.
As part of a previous project, meanwhile, Westray had written a simulator that generated realistic market orders. Over lunch, the two realised they could combine their ideas to put together an experiment.
“I told Kevin about the simulator, and he said it was exactly what he needed,” says Westray. “A way to generate lots and lots of orders that we could use to prove the efficacy of his method. After we talked, I realised we had all the ingredients to write a short but quite a nice paper.”
In that paper, Getting more for less: better A/B testing via causal regularisation, Webster and Westray demonstrate a more efficient means for quants to overcome bias in the data they use for transaction cost analysis (TCA).
The two quants took an algorithm developed by Dominik Janzing at Amazon in 2019 and applied it to simulated equities transaction data, showing how practitioners might debias transaction cost data at as little as a fifth of the cost.
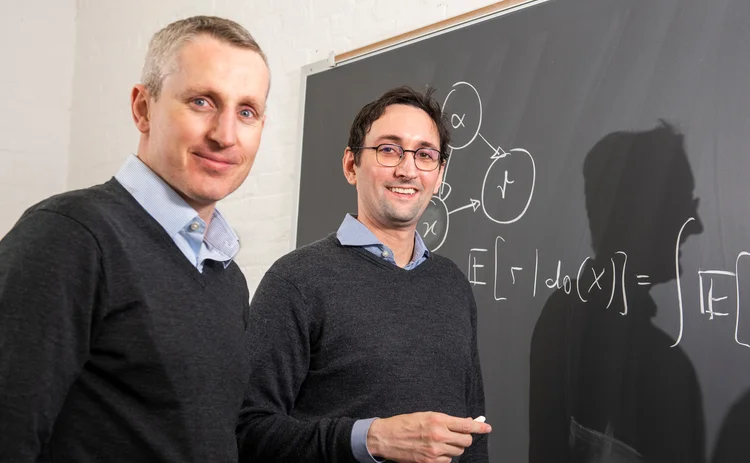
“Understanding these biases is a key part of our toolkit in diagnosing and solving the problems we face daily,” Westray says. “This forms part of that.”
Westray is the head of execution research in AllianceBernstein’s hedge fund and multi-asset solutions group, and visiting researcher in financial machine learning at New York University’s Courant Institute of Mathematical Sciences.
Webster is today a quantitative researcher at DE Shaw and until earlier this year was an adjunct assistant professor at Columbia University and visiting reader at Imperial College London.
Debiasing TCA data has been largely ignored in academic research. Practitioners see it as a crucial skill, though. (Westray declines to comment on whether AllianceBernstein has deployed the approach set out in the paper in its live trading.)
Correcting bias
The problem facing investors when they ask how their own trading may have moved prices, is to determine how prices might have moved anyway.
The data is unavoidably slanted. For a start, investors trade at times when they expect markets to move. Meanwhile, others are likely to be trading in similar ways, at similar times, and they, too, move prices.
Practitioners face a “classic garbage in garbage out problem”, Webster says – feeding into market impact models data that is likely to be misleading.
Pristine unbiased data is the best, but it’s the most expensive
Kevin Webster
To address the unwanted effects, investors carry out random test trades to provide a benchmark against which to measure their real trades – a process known as A/B testing.
Those tests, however, can be costly and time consuming. Capital Fund Management ran one such test for a year. “Pristine unbiased data is the best, but it’s the most expensive,” Webster says. “And not everybody has it or has a large quantity of it.”
Webster and Westray’s innovation has been to use a small subset of unbiased data as a reference point against which to debias a larger body of less reliable data.
The technique, known as causal regularisation, is a type of so-called transfer learning. A machine learning engine trains on both sets of data, and uses the unbiased set effectively to fine tune what it learns.
The two quants showed in their simulation that a trading experiment with 250 randomised trades that employed the new approach was superior to a standard A/B test with 1,250 randomised trades.
Their method leads to a model that is both more accurate and more reliable. Training on large but biased datasets, by contrast, leads to models that diverge further from reality. Training on unbiased but small samples leads to models with a large statistical margin of error.
“Very few people ever think about using machine learning in the market microstructure space,” says Yin Luo, vice-chairman at Wolfe Research, who was Webster’s boss while in a previous role Deutsche Bank. “That’s their biggest contribution.” Understanding market impact has gained importance for investors as alpha has become harder to generate, he adds.
Execution research
Both Westray and Webster have been interested in market microstructure for years.
After finishing his PhD in late 2008 — “not a particularly good time to be looking for a job in finance” — Westray took a position with a research institute in Berlin funded by Deutsche Bank, before moving to the bank itself two years later.
That role brought him into contact with Deutsche’s quants working on algorithmic trading. “I was working a lot with groups in London, New York and Hong Kong, asking questions about how to schedule trading, which tied in with the stochastic control research I’d been doing as a PhD student,” Westray says.
One of those individuals was Daniel Nehren, now at the Abu Dhabi Investment Authority, who in early 2015 joined Citadel to lead an equity execution group and approached Westray to join his team.
Understanding these biases is a key part of our toolkit in diagnosing and solving the problems we face daily
NIcholas Westray
Webster’s interest in market microstructure, meanwhile, goes as far back as his undergraduate studies at the Ecole Polytechnique, where he took classes led by Jean-Philippe Bouchaud, among others. Bouchaud is the chairman and head of research at Capital Fund Management and a leading expert on market impact.
In 2014, Webster started at Deutsche Bank in sell-side equity research under Luo, working mainly on transaction costs and market impact associated with the team’s alpha strategies. Then he, too, moved to Citadel, joining the same execution team as Westray in early 2016.
The two worked together for the next three years until Westray left Citadel in 2019.
Both used non-compete periods before taking up their most recent positions to complete academic work in the field. Westray authored several papers with NYU Courant’s Petter Kolm, including on the application of machine learning to order flow data.
He joined AllianceBernstein’s multi-asset solutions group in early 2021 in equities execution research, subsequently taking a broader role across asset classes and execution for the firm’s internal hedge fund.
Webster left Citadel at the end of 2021, since when he has written a book on price impact modelling and worked on half a dozen papers alongside co-authors including Johannes Muhle-Karbe, head of mathematical finance at Imperial College London, Marcel Nutz, a professor at Columbia, Bouchaud and Westray.
Webster joined DE Shaw this month, also in a role working on equities execution research.
Golden age
Transaction cost analysis may be entering its golden age, Westray says. The seminal paper on TCA by Robert Almgren and Neill Chris, Optimal execution of portfolio transactions, was published in 2001, but it is only in the past decade that this field of research has made significant advances.
The electrification and algorithmic trading of equities, futures and foreign exchange provides the data quants need to tackle market impact problems better. And machine learning gives practitioners a new set of tools.
“Market impact mis-specification can lead to disastrous results,” says CFM’s Bouchaud. It can cause investors to trade too aggressively and so move markets against themselves – or not aggressively enough and therefore to leave profits unrealised.
The quants say more can be done to draw on the techniques being developed in the tech industry. Both tech and finance enjoy an abundance of data, but none – or very little of it – is clean, Webster points out.
He recalls the mission statement of a tech firm statistics team that aimed simply to run experiments that its managers would have confidence in. He recognised the sentiment immediately, he says. “The main reason people distrust TCA reports is because biases are abundant in our field.”
Many of the data problems tech engineers are thinking hard about are causal in nature, he adds, a feature common in finance too. “As you increase sample size, the bias does not disappear.”
Westray adds that experiments similar to quants’ A/B testing are happening continuously in tech. “Amazon and Google run massive tests all the time for jobs such as targeting advertisements based on user behaviour,” he says. “They’re thinking very hard about how to organise large-scale experiments.”
Only users who have a paid subscription or are part of a corporate subscription are able to print or copy content.
To access these options, along with all other subscription benefits, please contact info@risk.net or view our subscription options here: http://subscriptions.risk.net/subscribe
You are currently unable to print this content. Please contact info@risk.net to find out more.
You are currently unable to copy this content. Please contact info@risk.net to find out more.
Copyright Infopro Digital Limited. All rights reserved.
You may share this content using our article tools. Printing this content is for the sole use of the Authorised User (named subscriber), as outlined in our terms and conditions - https://www.infopro-insight.com/terms-conditions/insight-subscriptions/
If you would like to purchase additional rights please email info@risk.net
Copyright Infopro Digital Limited. All rights reserved.
You may share this content using our article tools. Copying this content is for the sole use of the Authorised User (named subscriber), as outlined in our terms and conditions - https://www.infopro-insight.com/terms-conditions/insight-subscriptions/
If you would like to purchase additional rights please email info@risk.net
More on Awards
Joining the dots: banks leverage tech advancements for the future of regulatory reporting
The continued evolution of regulatory frameworks is creating mounting challenges for capital markets firms in achieving comprehensive and cost-effectiveawa compliance reporting. Regnology discusses how firms are starting to use a synthesis of emerging…
Markets Technology Awards 2024 winners' review
Vendors spy opportunity in demystifying and democratising – opening up markets and methods to new users
Derivatives house of the year: JP Morgan
Risk Awards 2024: Response to regional banking crisis went far beyond First Republic
Risk Awards 2024: The winners
JP Morgan wins derivatives house, lifetime award for El Karoui, Barclays wins rates
Best product for capital markets: Murex
Asia Risk Awards 2023
Technology vendor of the year: Murex
Asia Risk Awards 2023
Best structured products support system: Murex
Asia Risk Awards 2023
Energy Risk Asia Awards 2023: the winners
Winning firms demonstrate resiliency and robust risk management amid testing times
Most read
- Breaking out of the cells: banks’ long goodbye to spreadsheets
- Too soon to say good riddance to banks’ public enemy number one
- Industry calls for major rethink of Basel III rules