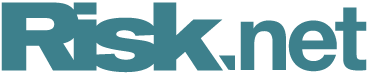
Risk data analytics house of the year: Shandong City Commercial Banks Alliance
Asia Risk Awards 2018

In 2016, after an assessment of their credit risk practices and tools, a group of 10 Chinese banks concluded they needed to join together to spruce up their defence against possible defaults.
Now, the banks are on the verge of launching a non-retail internal credit-rating project. With the support of Shandong City Commercial Banks Alliance (SCCBA), the lenders have managed to solve the biggest problems of assessing the credit risk of banks in China: limited data and insufficient modelling experience. The project also boasts artificial intelligence that combs through internal and external data.
Supervised by SCCBA, the project team consisted of SCCBA, Deloitte and 10 commercial banks, which have combined assets under management of 1 trillion yuan ($150 billion): Chang’an Bank, DeZhou Bank, DongYing Bank, LinShang Bank, Bank of JiNing, QiShang Bank, Bank of RiZhao, Bank of WeiFang, YanTai Bank and Taian Bank. After 18 months, 30 models had been finalised by early 2018.
A test run has already shown the potential of the project. For instance, one member bank found that if the model had been applied five years earlier, a total of 401 client defaults, amounting to 4.2 billion yuan ($600 million) in losses, could have been identified in advance. With non-performing loans on the rise – they jumped 183 billion yuan to 1.96 trillion yuan in the three months leading up to June, which was the biggest quarterly jump in data going back more than a decade, according to data from the China Banking and Insurance Regulatory Commission (CBIRC) – such early warnings are bound to prove their worth.
“In the past five to 10 years, bank loan defaults in China have increased dramatically, so banks needed to invest in a better system. By doing it with 10 banks, we can tap multiple experts in data,” says Chang Kun, general manager of the department of innovation and co-operation at SCCBA.

The genesis of the project can be traced to the joint implementation of Basel rules by SCCBA and member banks a few years ago, under the guidance of the CBIRC Shandong bureau. The aim was to improve the risk management capability of city commercial banks.
This began in September 2016, and over the next three months the team extracted data from the 10 banks with the help of thousands of account managers. It then developed a data-replenishing platform with built-in automatic verification rules to monitor replenishment progress and quality in real time.
While the data of a single bank was insufficient, the tests proved combining the data and experience of the 10 banks gave very robust results. The team combined the four dimensions of index selection, index weight setting, index column division and column corresponding score. This gave it an edge on previous non-retail internal credit rating projects, which mainly relied on expert experience.
The project also came armed with an integrated artificial intelligence technology that stepped beyond internal data to analyse various sources of news, public filings such as tax reports, customs, judicial data and declarations with utilities and government authorities, and compared them with a company’s own financial statements. It can also compare the rating results with a public opinion analysis algorithm, jointly developed by Deloitte and the Chinese Academy of Sciences to fully ensure the objectivity and accuracy of the ratings for different business scenarios and industry characteristics.
The new technology also aims to solve the common problems in verifying and auditing the authenticity of financial statements, including potential forgery. It uses the data from the 10 banks to analyse their business situation item by item, then forms a complete set of checkout tools for financial statements.
“The project has strengthened the management framework of the internal evaluation system, developed a set of models and executed these to the credit management process system, which will effectively improve the member banks’ risk management ability,” notes an emailed statement from CBIRC.
SCCBA believes the project not only enhances the application of quantitative models, but also makes breakthroughs in the use of big data scenarios, artificial intelligence and other technologies.
A risk manager at one of the member banks says the project improved the accuracy and stability of the risk management function of the lender, and also helped it comply with regulatory capital rules.

With the evolution of data and analytical tools, innovation is a key objective of the SCCBA project team. “There are quite a few internal credit ratings projects out there, but this is probably the only one to use AI, big data analytics and other bleeding edge technology in this project,” says Jerry Yu, a partner at Deloitte RA.
Member banks are aware of the benefits of data analytics and AI to the project. One official says, “artificial intelligence technology, combining the results of ratings with a public opinion analysis algorithm, ensures the rating results are objective and accurate. It should be the popular choice for the whole country, especially for banks that lack data samples”.
About 95% of the project implementation has been completed, and it is now going live across member banks. They are now trying to pin down a new business model, in order to keep improving non-retail models and the efficiency of credit risk management.
“This project is the largest non-retail internal rating project involving multi-corporate institutions of member banks in China,” says Shi Yuefeng, chairman of the board of the banking alliance. “The problem of data shortage and the inexperience of single firms has been solved successfully through this project. It is something that should be rolled out to other small and medium-sized banks.”
Only users who have a paid subscription or are part of a corporate subscription are able to print or copy content.
To access these options, along with all other subscription benefits, please contact info@risk.net or view our subscription options here: http://subscriptions.risk.net/subscribe
You are currently unable to print this content. Please contact info@risk.net to find out more.
You are currently unable to copy this content. Please contact info@risk.net to find out more.
Copyright Infopro Digital Limited. All rights reserved.
As outlined in our terms and conditions, https://www.infopro-digital.com/terms-and-conditions/subscriptions/ (point 2.4), printing is limited to a single copy.
If you would like to purchase additional rights please email info@risk.net
Copyright Infopro Digital Limited. All rights reserved.
You may share this content using our article tools. As outlined in our terms and conditions, https://www.infopro-digital.com/terms-and-conditions/subscriptions/ (clause 2.4), an Authorised User may only make one copy of the materials for their own personal use. You must also comply with the restrictions in clause 2.5.
If you would like to purchase additional rights please email info@risk.net
More on Awards
Counterparty risk innovation of the year: Cumulus9
Cumulus9 proved standout vendor by bringing inventive solutions to the market, securing the award for Counterparty risk innovation of the year at the Risk Technology Awards 2024
Life and pensions ALM system of the year: Conning
Conning proved to be a standout vendor in the life insurance and pensions space and secured the Life and pensions ALM system of the year award at the Risk Technology Awards 2024
Joining the dots: banks leverage tech advancements for the future of regulatory reporting
The continued evolution of regulatory frameworks is creating mounting challenges for capital markets firms in achieving comprehensive and cost-effectiveawa compliance reporting. Regnology discusses how firms are starting to use a synthesis of emerging…
Markets Technology Awards 2024 winners' review
Vendors spy opportunity in demystifying and democratising – opening up markets and methods to new users
Derivatives house of the year: JP Morgan
Risk Awards 2024: Response to regional banking crisis went far beyond First Republic
Risk Awards 2024: The winners
JP Morgan wins derivatives house, lifetime award for El Karoui, Barclays wins rates
Best product for capital markets: Murex
Asia Risk Awards 2023
Technology vendor of the year: Murex
Asia Risk Awards 2023